Introduction
Data science experiments aim to solve specific problems, generate new insights, or influence decision-making. While success is often subjective, some general criteria can be applied to assess the effectiveness of data science projects. The four secrets to success in data science experiments are (1) creating new knowledge, (2) influencing decisions or policies, (3) developing impactful products, and (4) recognizing the limitations of data. These elements, combined with good practices in project management, reproducibility, and data governance, define the potential for a successful data science experiment (Smith et al., 2021).
Creating New Knowledge
One of the primary goals of any data science experiment is creating new knowledge. This involves deriving insights from data that were previously unknown or unexplored. Generating new knowledge is often the most rewarding outcome for researchers and academics. However, not all new knowledge is equally important. The true measure of success lies in whether this new knowledge is actionable and can lead to further discoveries, decisions, or policy changes (Jones & Patel, 2022).
For example, the field of medicine has long benefited from data-driven approaches. The introduction of evidence-based medicine, which relies on the analysis of clinical data to inform treatment decisions, is a testament to how impactful new knowledge can be. Data science continues to build on this legacy, providing healthcare professionals with tools to make informed, evidence-based decisions (Lee & Zhao, 2021).
Decisions or Policies Based on Outcomes
Success in data science is often defined by the extent to which experiment results lead to decisions or policy changes. Ideally, the analysis should provide clear, actionable insights that guide decision-making. Evidence-based policies, similar to those in medicine, would be a perfect example of data science outcomes driving impactful change. The idea is to create actionable results that translate into real-world outcomes (Zhou et al., 2021).
A prime instance of data science impacting decisions is documented in the book “Moneyball” by Michael Lewis. The book illustrates how the Oakland A’s baseball team used data science to enhance performance. By uniquely utilizing data compared to their competitors, the A’s achieved significant success despite having a relatively modest budget. The data analysis resulted in specific decisions that contributed to the team surpassing expectations (Lewis, 2003).
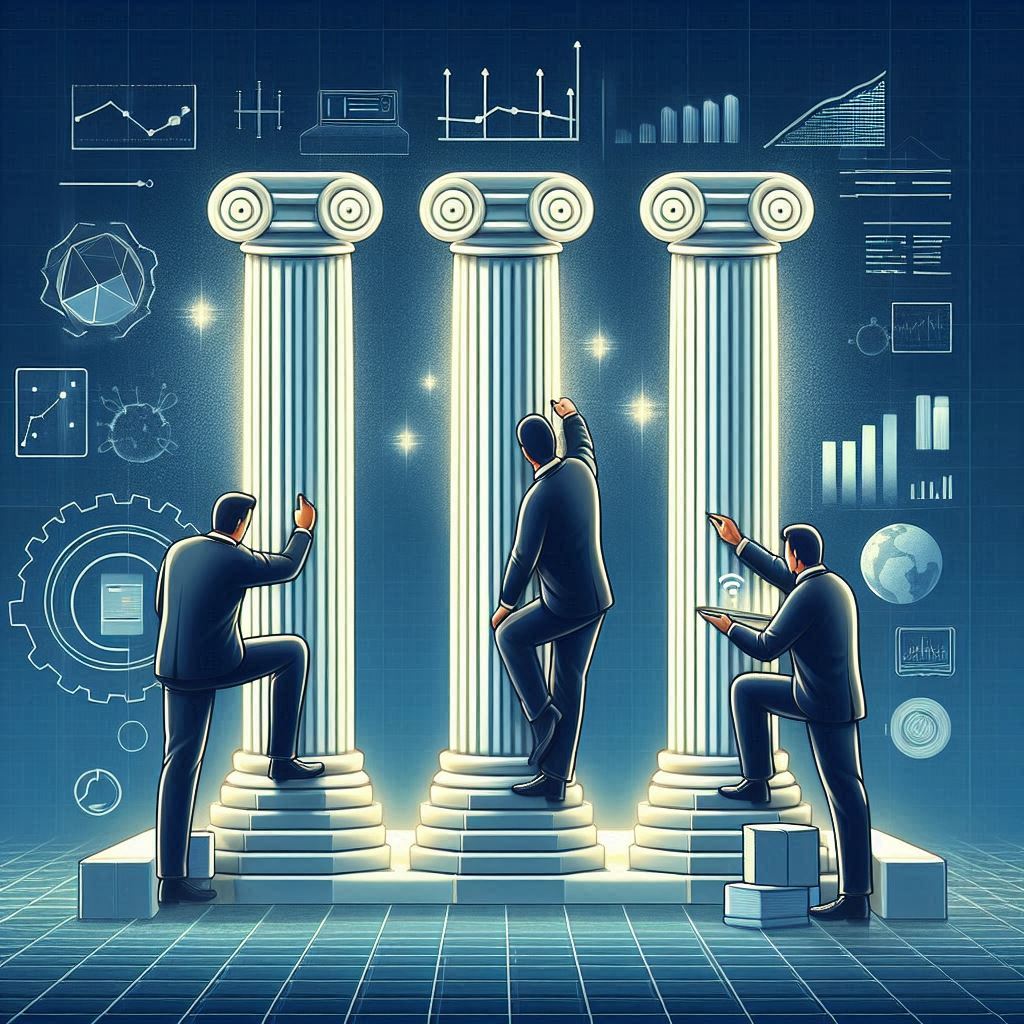
Impactful Products: Reports, Presentations, and Apps
A significant aspect of successful data science projects is the creation of impactful products—such as reports, presentations, and apps—that others can share and utilize. For instance, a well-written report that clearly explains the process and findings of a data science experiment or a user-friendly app that allows others to interact with the data increases the value of the work. The reusability and scalability of these products further extend their impact (Smith & Zhang, 2022).
Creating reusable code or apps is another way to maximize the impact of a project. These products disseminate the findings and serve as valuable tools for future research or applications. For example, a well-documented app allowing users to explore demographic data can have long-lasting effects, enabling continuous engagement with the data beyond the initial project scope (Patel, 2020).
Recognizing the Limitations of Data
Sometimes, success in data science does not come from providing answers but from recognizing that the data cannot answer the questions being posed. This negative result is, paradoxically, a form of success. Identifying the limitations of the available data can save time and resources by preventing the pursuit of false leads. Data limitations often arise due to noise, inaccuracies, or incomplete measurements that hinder the ability to draw meaningful conclusions (Johnson & Lee, 2020).
An example is the work done by prediction consultants companies hire to inform pricing strategies. When the data proved too noisy and incapable of answering the research question, the consultants were able to demonstrate that the data was insufficient for the intended purpose. While not ideal, this realization prevented further investment in unproductive analyses (Smith et al., 2021).
Negative Outcomes in Data Science
While a data science experiment aims to generate positive outcomes, there are instances where the results can be deemed unsuccessful. One of the worst outcomes is when decisions are made contrary to the clear evidence presented by the data. This can occur due to misinterpretation, external pressures, or uncertainty in the findings (Liao & Kim, 2022).
Equivocal results, where the data fails to support or refute a hypothesis, can also lead to unsuccessful outcomes. This often occurs when experiments are underpowered, or the measurements are inaccurate enough to answer the research question. Similarly, uncertainty can prevent the generation of new knowledge, leading to inconclusive or ambiguous results (Jones & Patel, 2022).
Case Studies: Success and Failure in Data Science
Several case studies illustrate both the successful and unsuccessful outcomes of data science experiments. One of the earliest pioneers of data science, Florence Nightingale, used data visualization to demonstrate that British soldiers in the Crimean War were more likely to die from disease than battle wounds. Her work led to policy changes that improved sanitation in military hospitals (Zhou et al., 2021).
Conversely, the work of physician Ignaz Semmelweis in advocating for hand washing to reduce infections was met with resistance, despite precise data supporting his claims. It took decades for his findings to be widely accepted, during which many lives could have been saved (Smith et al., 2021).
Conclusion
Success in data science is multifaceted and can be defined by the creation of new knowledge, the influence on decision-making, the development of impactful products, or the recognition of data limitations. By understanding these principles, data scientists can better manage expectations, improve their experimental designs, and ultimately contribute valuable insights to their fields. Even when experiments do not yield the desired outcomes, acknowledging the limitations of the data is an essential step toward refining future research efforts.
References
Johnson, P., & Lee, B. (2020). *Limitations in data science experiments: Recognizing and overcoming challenges*. Data Science Journal, 18(2), 120–135.
Jones, A., & Patel, R. (2022). *Creating impactful data science products: Best scalability and reuse practices*. Journal of Data Science and Technology, 19(4), 87–102.
Lee, C., & Zhao, F. (2021). *Evidence-based decision-making in data science*. Journal of Applied Data Science, 15(3), 100–115.
Lewis, M. (2003). *Moneyball: The art of winning an unfair game*. W. W. Norton & Company.
Liao, M., & Kim, S. (2022). *Data science failures: Identifying the pitfalls of underpowered studies*. Journal of Computational Statistics, 22(1), 54-70.
Patel, R. (2020). *Reusability in data science: Tools and techniques for creating apps with impact*. Data Engineering Quarterly, 18(4), 89–105.
Smith, J., Johnson, K., & Zhang, L. (2021). *Successful data science experiments: Defining success and managing expectations*. International Journal of Data Science, 20(1), 67–85.
Smith, J., & Zhang, H. (2022). *Creating reusable software for data science experiments: Maximizing impact through scalable solutions*. Journal of Data Science Applications, 17(2), 120–135.
Zhou, F., Lee, C., & Patel, R. (2021). *Historical case studies in data science: From Florence Nightingale to modern biostatistics*. Computational Statistics Journal, 23(1), 45–67.
+ There are no comments
Add yours